Mathematical theory of deep learning
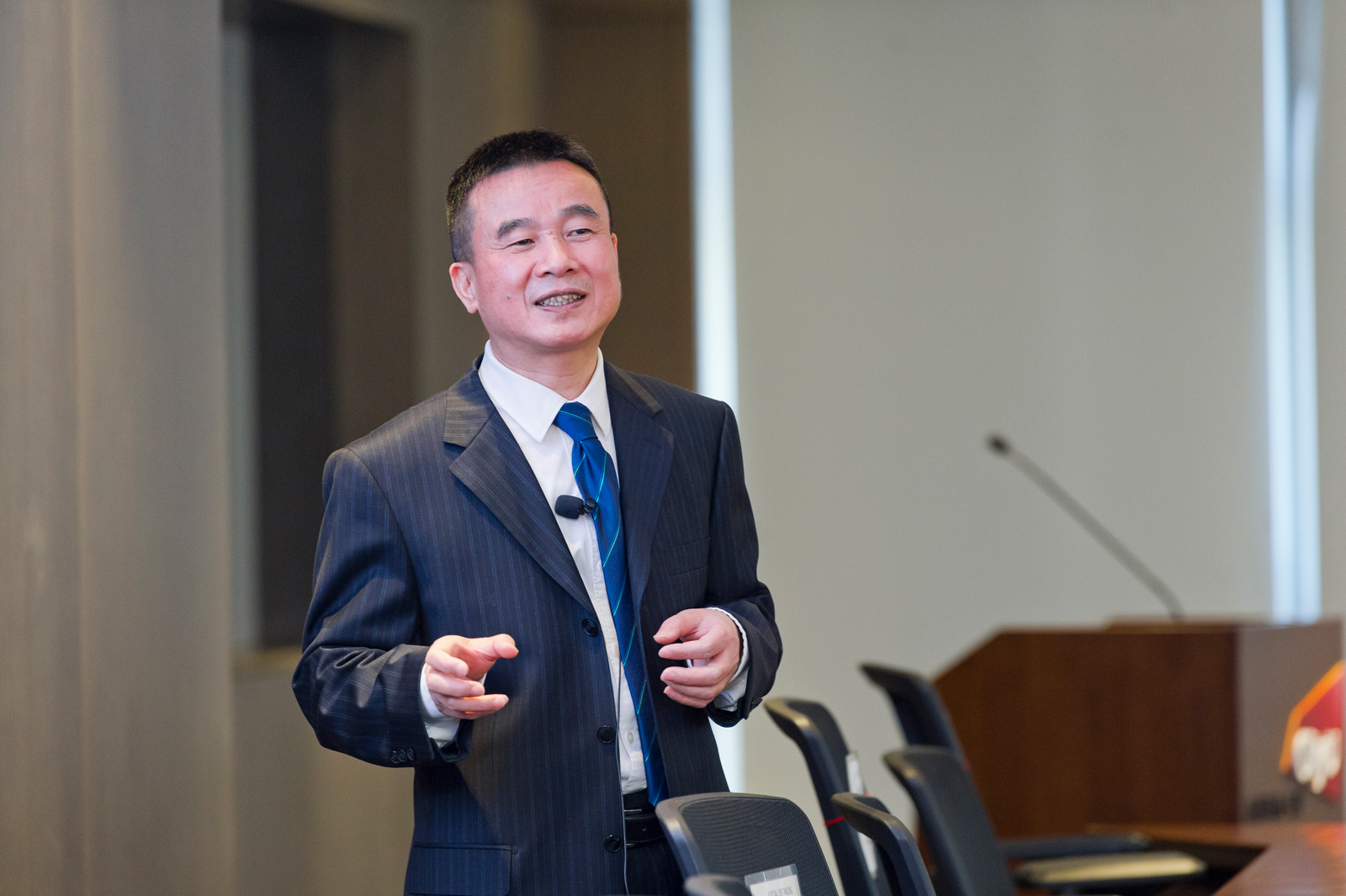
Deep learning has resulted in breakthroughs in dealing with big data, speech recognition, computer vision, natural language processing, and many other domains, according to Professor Zhou Dingxuan at the 46th talk in the President’s Lecture Series: Excellence in Academia at CityU University of Hong Kong (CityU) on 11 November.
But is there a mathematical theory to help us to better understand deep learning? That was the thesis embedded in a well-attended and well-received online talk titled “Mathematical theory of deep learning”.
Deep learning is a type of machine learning and AI that imitates the way humans gain certain types of knowledge.
“A mathematical foundation is needed to help understand the modelling and the approximation, or generalisation capability, of deep learning models with network architectures and structures,” explained Professor Zhou, Chair Professor and Associate Dean of the School of Data Science; Chair Professor of the Department of Mathematics; and Director of Liu Bie Ju Centre for Mathematical Sciences.
In this talk, Professor Zhou considered deep convolutional neural networks (CNNs) that are induced by convolution, explaining that convolutional architecture identifies essential differences between deep CNNs and classic neural networks.
The talk was conducted online and attracted scholars and experts from different parts of the world, as well as academics and students from CityU and other local universities.
Professor Zhou is listed among the top 2% of the world's most highly cited scientists, according to metrics compiled by Stanford University, and has been named as a Highly Cited Researcher by Clarivate Analytics. He is the Editor-in-Chief of the journals Analysis and Application and Mathematical Foundations of Computing, and serves on the editorial boards of more than 10 journals.