

Opportunity
Segmentation of medical images is necessary for the diagnosis and treatment of various diseases and medical conditions. Although the recent development of convolutional neural networks has led to interest in their use for image segmentation, their application is limited by the expensive and time-consuming requirement for large libraries of pixel-annotated training images. Another approach, weakly supervised segmentation using bounding-box annotation to generate proposals (i.e., “fake labels”), does not require annotated images and has shown promise, but its applicability may be limited by the complex shapes of organs and other biological structures and by imaging artifacts. An approach to weakly supervised segmentation of medical images that overcomes these limitations is needed.
Technology
Researchers have developed a computer-implemented method for segmenting a 3D medical image to produce multiple segmentation masks. First, three components are set up. The backbone is an artificial neural network (ANN) used to generate feature maps from an input 3D image. The mask head is a second ANN used to generate segmentation maps from the feature maps. The contrastive head is a third ANN used to map pixels from the feature maps to an embedding space, which is used to determine the positions of the pixels. This allows evaluation of the contrastive similarity between pixels.
All three components are trained using a set of 3D training images that have been subjected to bounding-box annotation; as a result, a bounding box is specified and an organ proposed. The mask head then uses a template organ to improve the contrast of the proposed organ against the background and segments the training image.
Advantages
- Reduces the need for a large training dataset
- Reduces the time and cost of computing
Applications
- Medical imaging
- Clinical diagnosis
- Research imaging

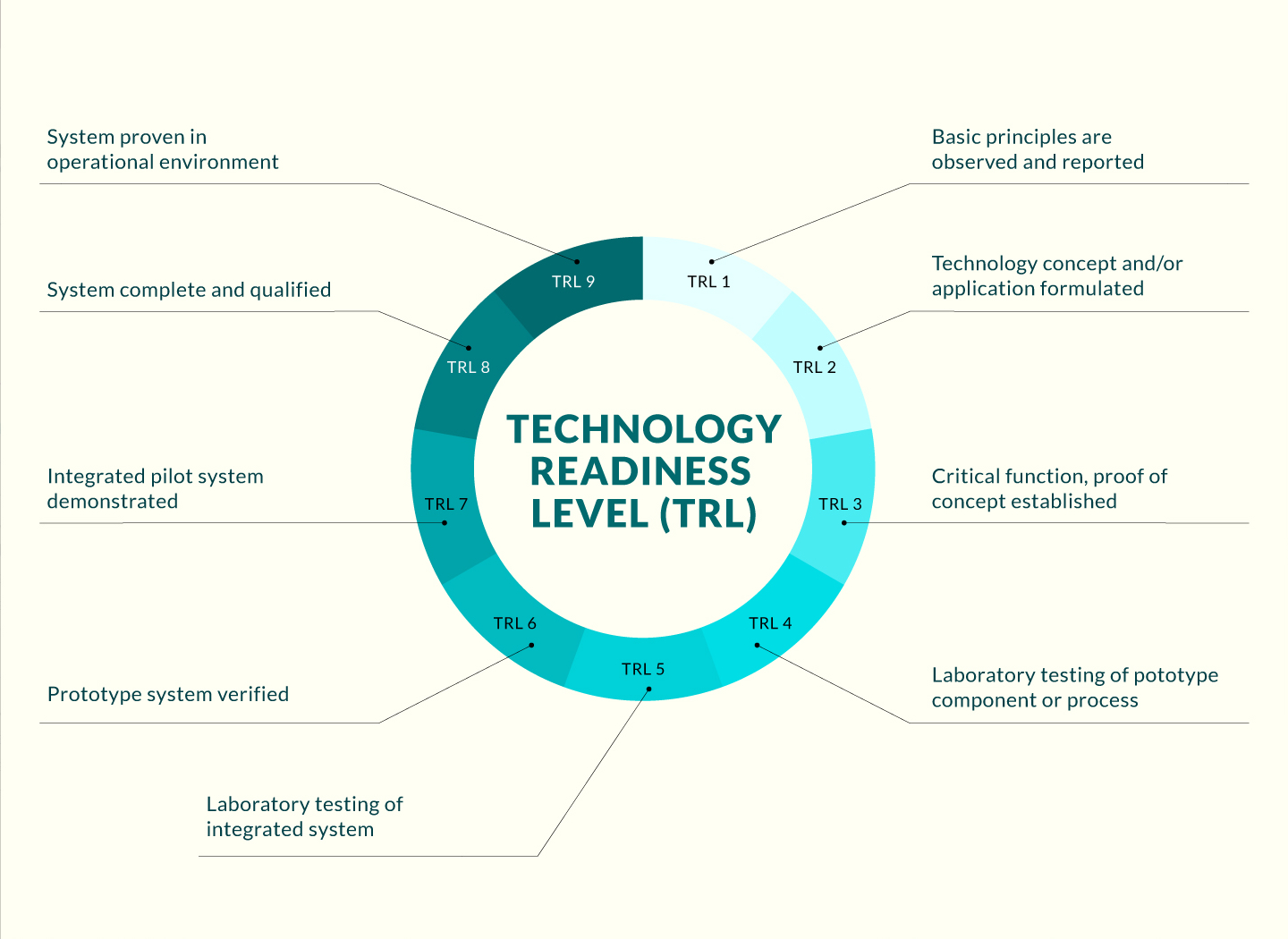
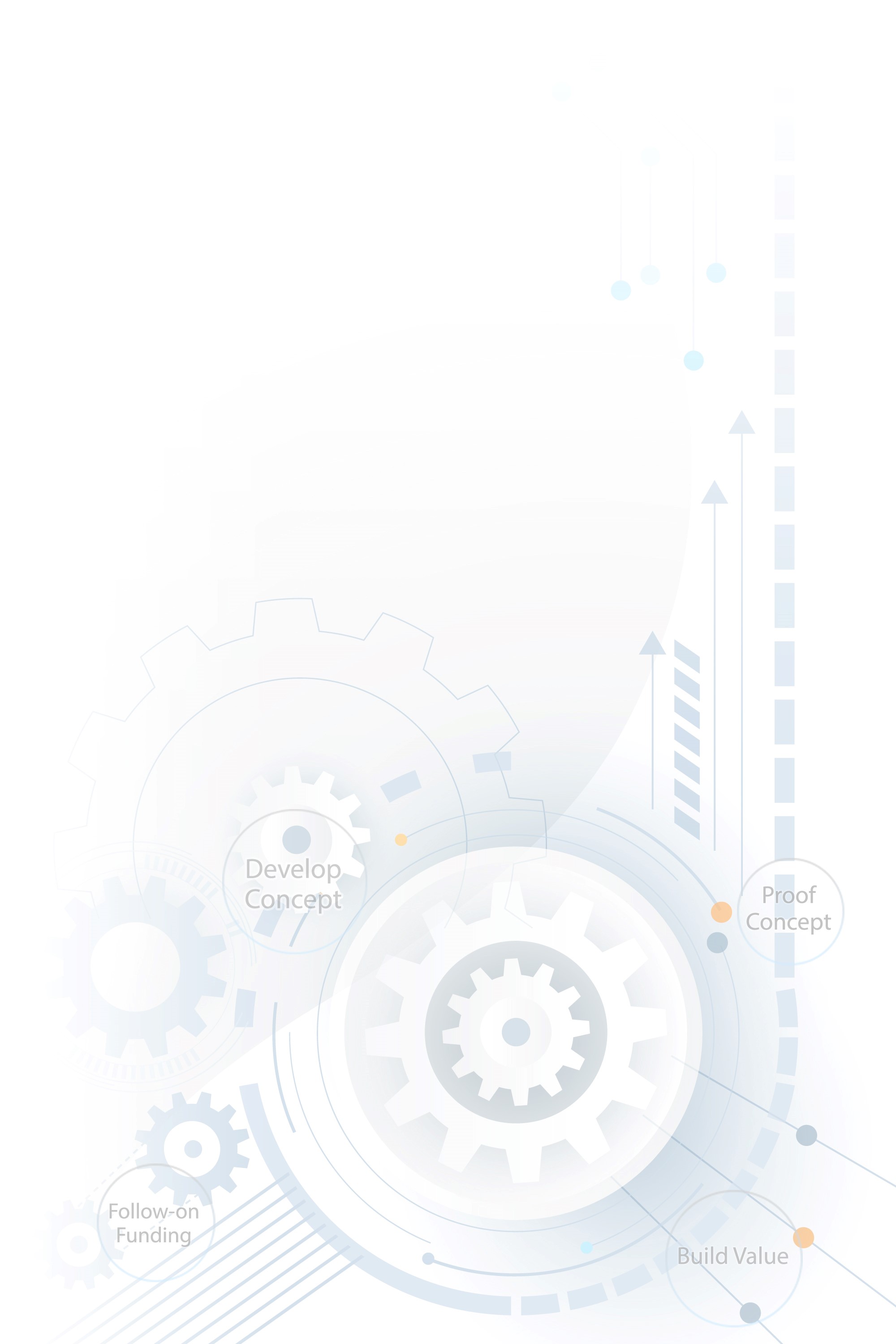