


Opportunity
Gaze tracking technique can be used to determine where the user is looking at by monitoring, in real-time, the eye motions of freely-moving users. The general challenges of 3D gaze estimation for head-mounted eye tracking systems are inflexible marker-based calibration procedure and significant errors of depth estimation.
Some high-end Mobile Head-mounted Gaze Trackers (HMGTs) address the said issues by employing fully calibrated geometrical systems, while for low-cost HMGTs the eyeball positions can be estimated by formulating a nonlinear optimization problem aims at the reduction of angular differences between the training gaze direction and prediction ones. However, those existing commonly-used unconstrained optimization may provide an erroneous eye position, which leads to unsatisfactory generalization capacities.
Technology
The present invention relates to a system and method for gaze estimation that includes processing training data and determining one or more local-learning base gaze estimation model based on the training data. The local-learning base gaze estimation model(s) can be used for determining one or both of: 2D gaze points in a scene image and 3D gaze points in scene camera coordinates.
To acquire the accurate 3D structure of the environment, an RGBD camera is applied as the scene camera of the system. By adopting the saliency detection method, saliency maps can be acquired through scene images, and 3D salient pixels in the scene are considered potential 3D calibration targets. The 3D eye model is built on the basis of eye images to determine gaze vectors. By combining 3D salient pixels and gaze vectors, the auto-calibration can be achieved with our calibration method. Finally, the 3D gaze point is obtained through the calibrated gaze vectors, and the point cloud is generated from the RGBD camera. It presents a great improvement in depth measurement, which is sufficient for tracking users' visual attention in real scenes.
Advantages
- It only requires the basic hardware setup with two eye cameras.
- For the 2-D gaze point estimation, the parallax and interpolation/extrapolation errors can be adequately compensated via designing a suitable regression model and training data acquisition process to collect an informative distribution of training data.
- For the 3-D gaze point estimation, the regression-based method can be used without the requirements of the knowledge of precise eyeball models and the calibration for the eye cameras.
- It can make eye tracking techniques accessible to eyewear users, which presents a significant portion of the entire population.
Applications
- Headset of Virtual Reality (VR) and Augment Reality (AR)
- Patients of Locked-in Syndrome

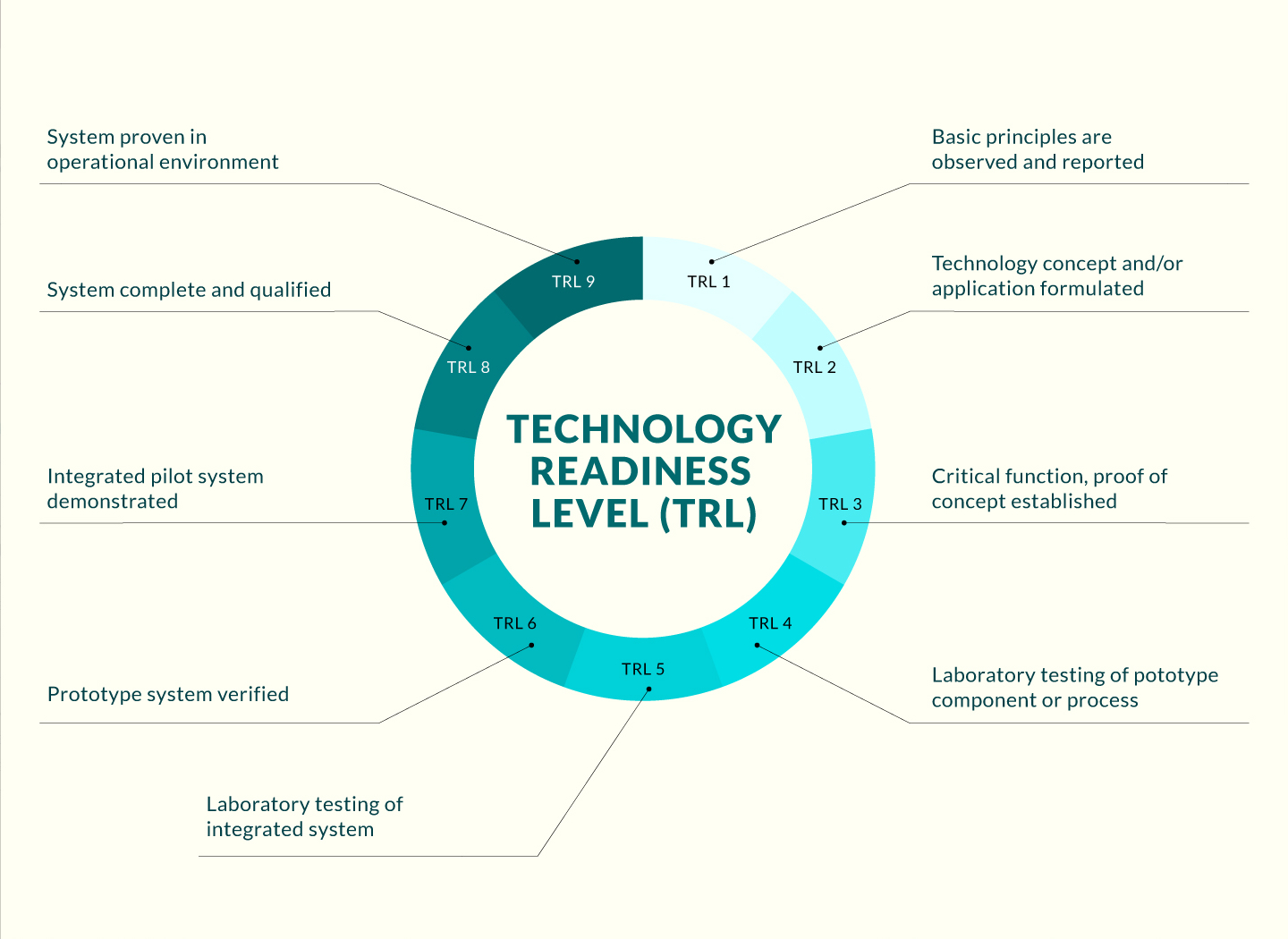
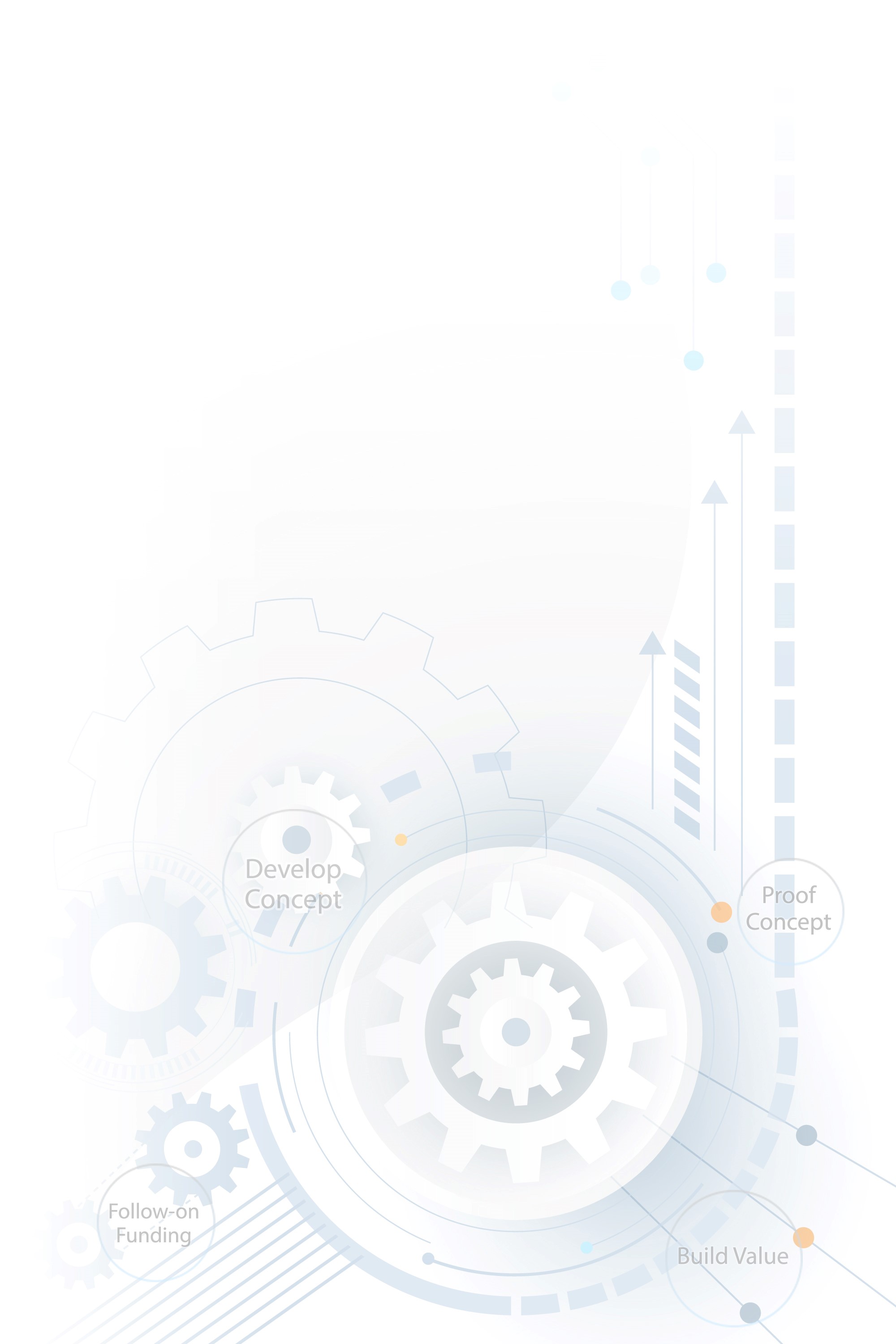