
Opportunity
The current recommendation systems face limitations in providing accurate and personalized recommendations, especially in large-scale and diverse user environments. The opportunity lies in the invention of a federated recommendation system. By leveraging a central server and client devices, this technology enables the generation of personalized recommendation models for each user within their respective clusters. This approach addresses the shortcomings of traditional recommendation systems and opens up new possibilities for companies operating across different geographical locations or user segments. The market opportunity involves refining the technology, highlighting its advantages over existing methods, and forming strategic partnerships with companies seeking to enhance their recommendation initiatives. A two-tiered SaaS business model, offering cluster-level and global-level services, combined with additional revenue streams such as consultation and support services, can help businesses capitalize on this technology.
Technology
The federated recommendation system is an advanced technology that improves the accuracy and personalization of recommendation systems. It works by using a central server and client devices to create personalized recommendation models for each user. The server groups users into clusters and processes their individual preferences using graph neural networks. It then combines these preferences to create a global model. The system provides both cluster-level and global-level models to the client devices, enabling them to generate personalized recommendations. This technology is beneficial for businesses operating in different locations or with diverse user segments, such as e-commerce platforms or streaming services, as it enhances the recommendation process and improves user satisfaction.
Advantages
- Enhanced Personalization: Our federated recommendation system generates personalized recommendation models for each user, resulting in more accurate and tailored recommendations.
- Scalability: The system can handle large-scale and diverse user environments, making it suitable for companies operating in various geographical locations or with different user segments.
- Improved Accuracy: By combining individual preferences from users within clusters, the system creates both cluster-level and global-level models, leading to more precise recommendations.
- Privacy Preservation: The federated learning approach ensures that user data remains on the client devices, preserving privacy while still providing personalized recommendations.
- Competitive Edge: By adopting our advanced technology, businesses can gain a competitive advantage by offering superior recommendation experiences, increasing user engagement, and driving revenue growth.
Applications
- E-commerce Platforms: Our recommendation system can be applied to e-commerce platforms, helping users discover relevant products and improving the shopping experience.
- Streaming Services: Our technology can enhance the content recommendation feature in streaming services, suggesting movies, TV shows, and music based on user preferences.
- News Portals: Our recommendation system can provide personalized news and article suggestions on news portals, ensuring users receive relevant and interesting content.
- Social Media Platforms: Our technology can be applied to social media platforms, offering personalized recommendations for users to connect with friends, join communities, and discover new content
- Online Marketplaces: Our recommendation system can enhance the browsing and shopping experience on online marketplaces, suggesting relevant products and increasing user engagement.

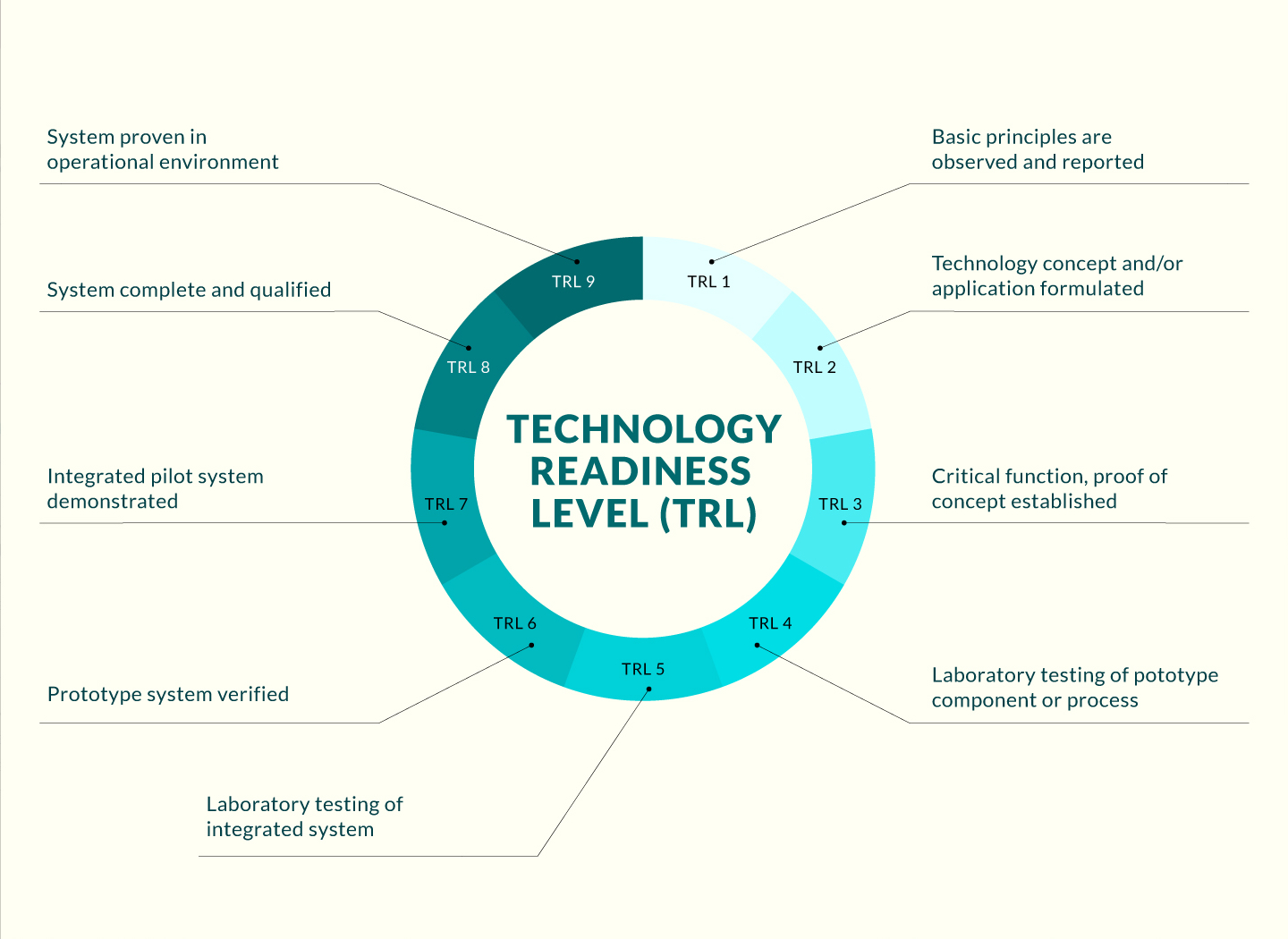
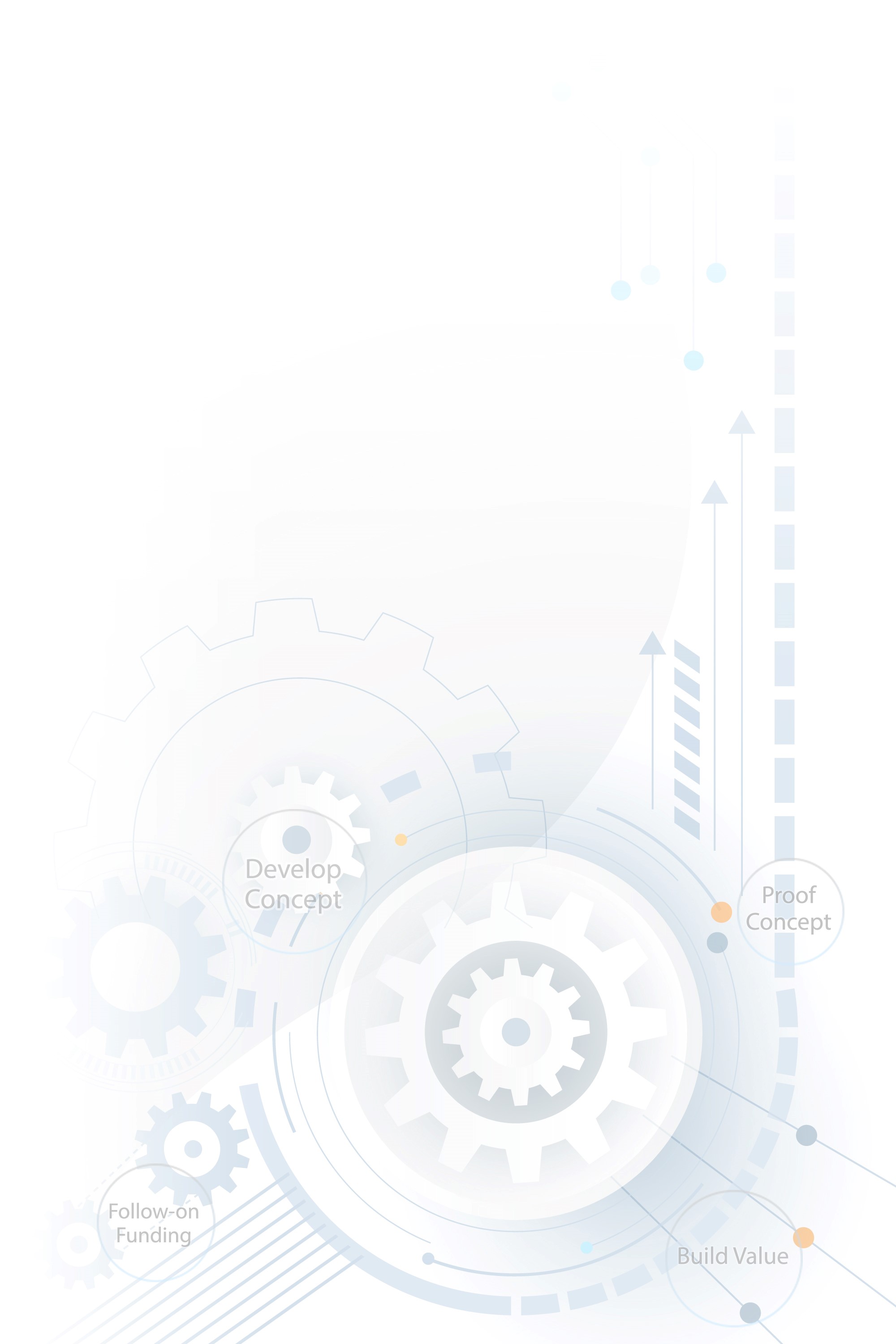